Why Is Building Energy Characterization Important?
Increasing Energy Use
Commercial buildings consume 35% of US electricity, 37% of which is used for heating, ventilation, air conditioning, and refrigeration. With the increase in global construction projects, the need for energy, including air conditioners and other appliances, is also growing.
Long Lifetimes
Buildings last a long time, and they don't get replaced or renovated quickly. Although there has been a focus on creating energy-efficient buildings, most commercial buildings already in use were constructed a long time ago, with the average construction year in the United States being 1981.
Climate Goals
Climate goals drive ambitious electrification targets, which involves transforming a significant portion of our existing energy infrastructure to rely more on electricity, and to reduce dependence on traditional sources that contribute to environmental issues.
What Does Building Energy Characterization Entail?
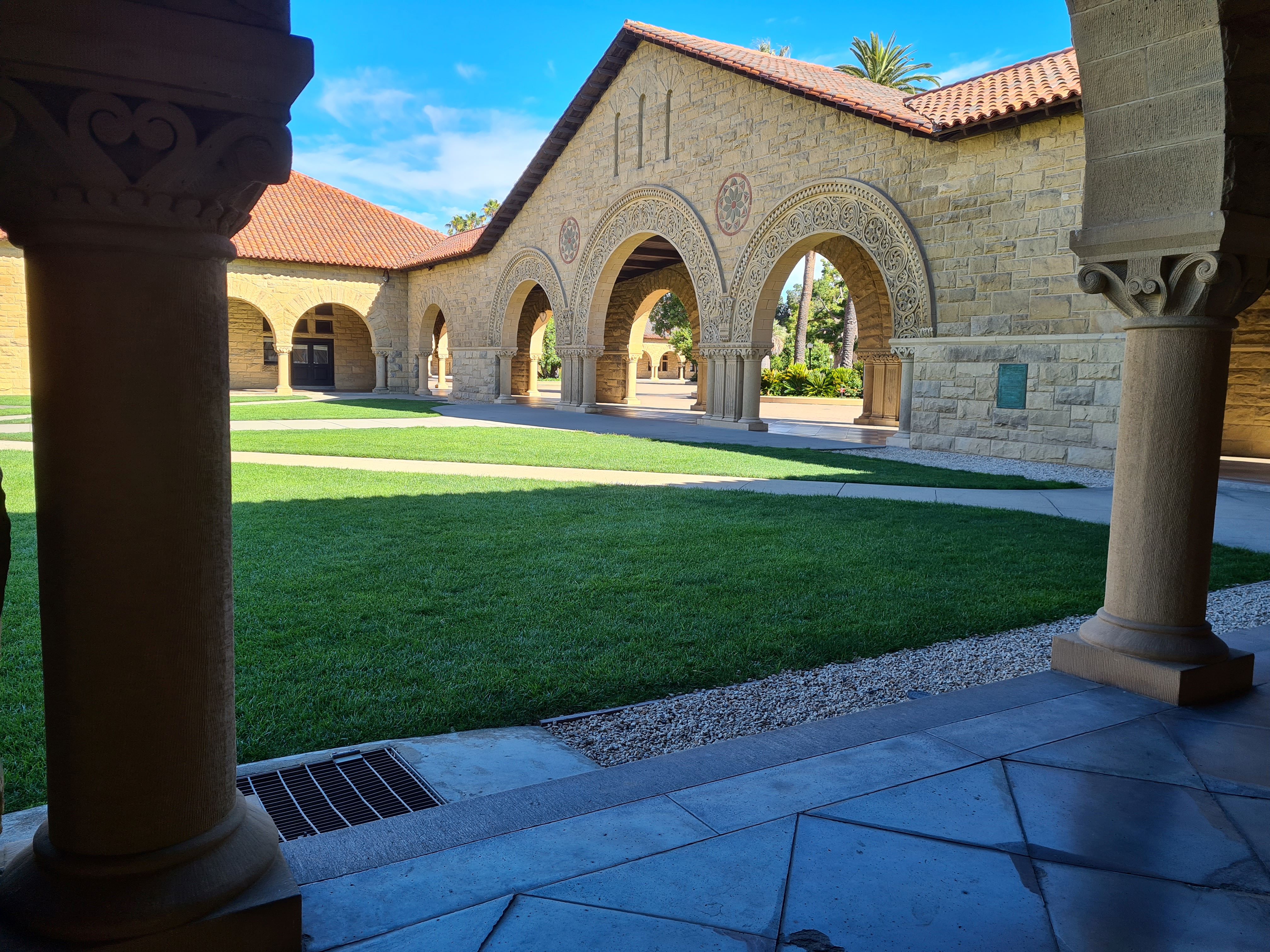
Data-driven Modeling
Explore the efficacy of data-driven models in characterizing the energy consumption (specifically, cooling) across diverse types of buildings.
Drivers of Energy Consumption
Investigate and identify the key factors influencing energy consumption in commercial buildings.
Performance Monitoring
Extract valuable lessons from the models to propose strategies and recommendations for enhancing the energy efficiency of commercial buildings.
Diversity in Buildings
Analyze and explain the variations in energy demands among co-located buildings, exploring the reasons behind the observed heterogeneity.
Strategy
EXPLORE, MODEL, ANALYZE
Data Exploration and Visualization
Analyze chilled water data from over a hundred co-located buildings in a warm Mediterranean climate. Uncover insights about summertime cooling usage across diverse commercial buildings.
Scalable, Interpretable Models
Leverage data-driven regression models to characterize cooling requirements of individual building. These models, designed for adaptability, identify influential features in cooling consumption.
Decomposition of Loads
The regression models break down cooling loads into two components: base load intensity and temperature sensitivity. This breakdown enables characterization of the differences in energy use among buildings.
Unlocking Hidden Value
Energy Analytics for Informed Decisions
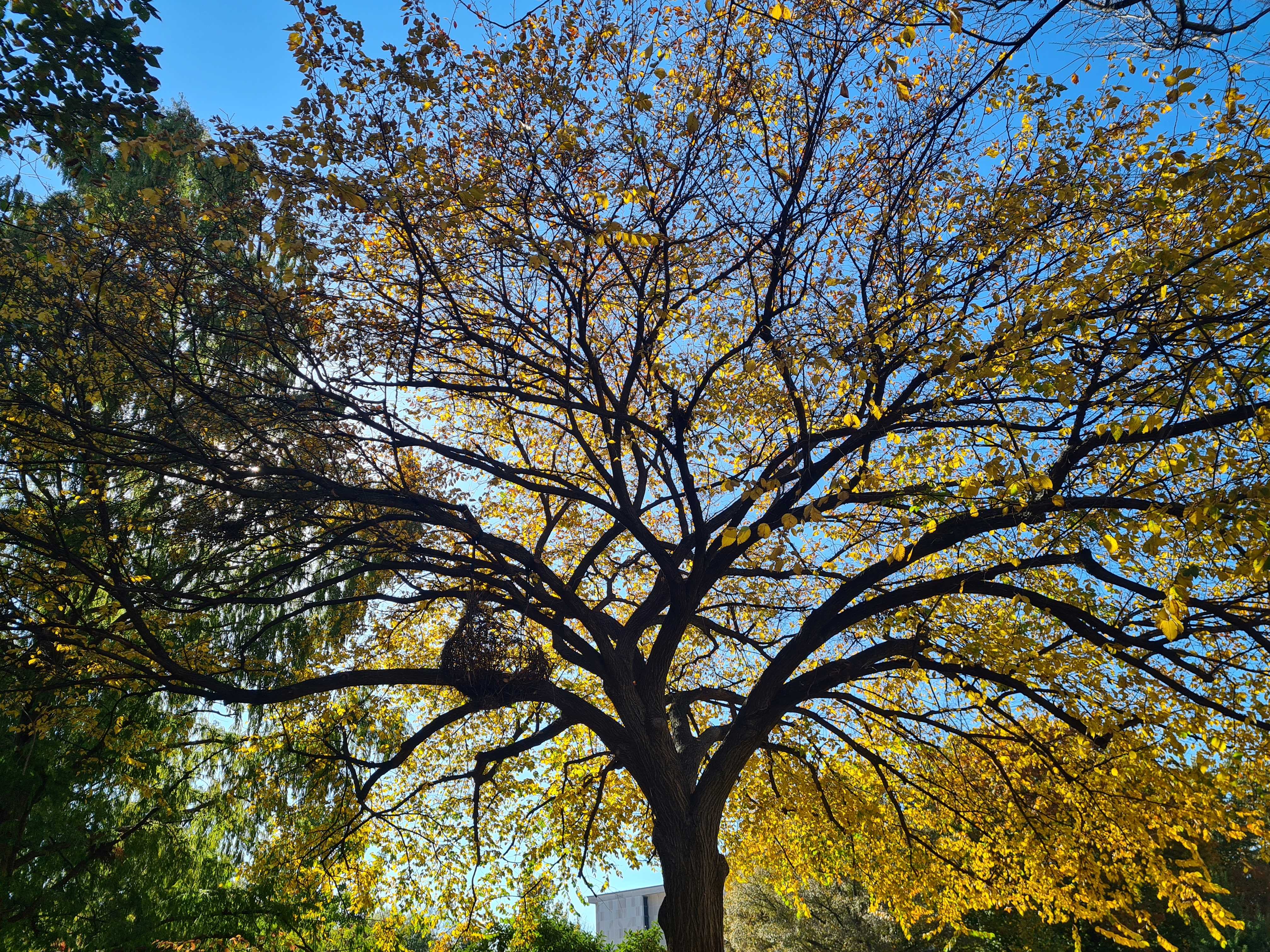
Data-Driven Baselines
Promptly forecast the future demand in response to warming temperatures, which is invaluable for planning and optimizing space conditioning.
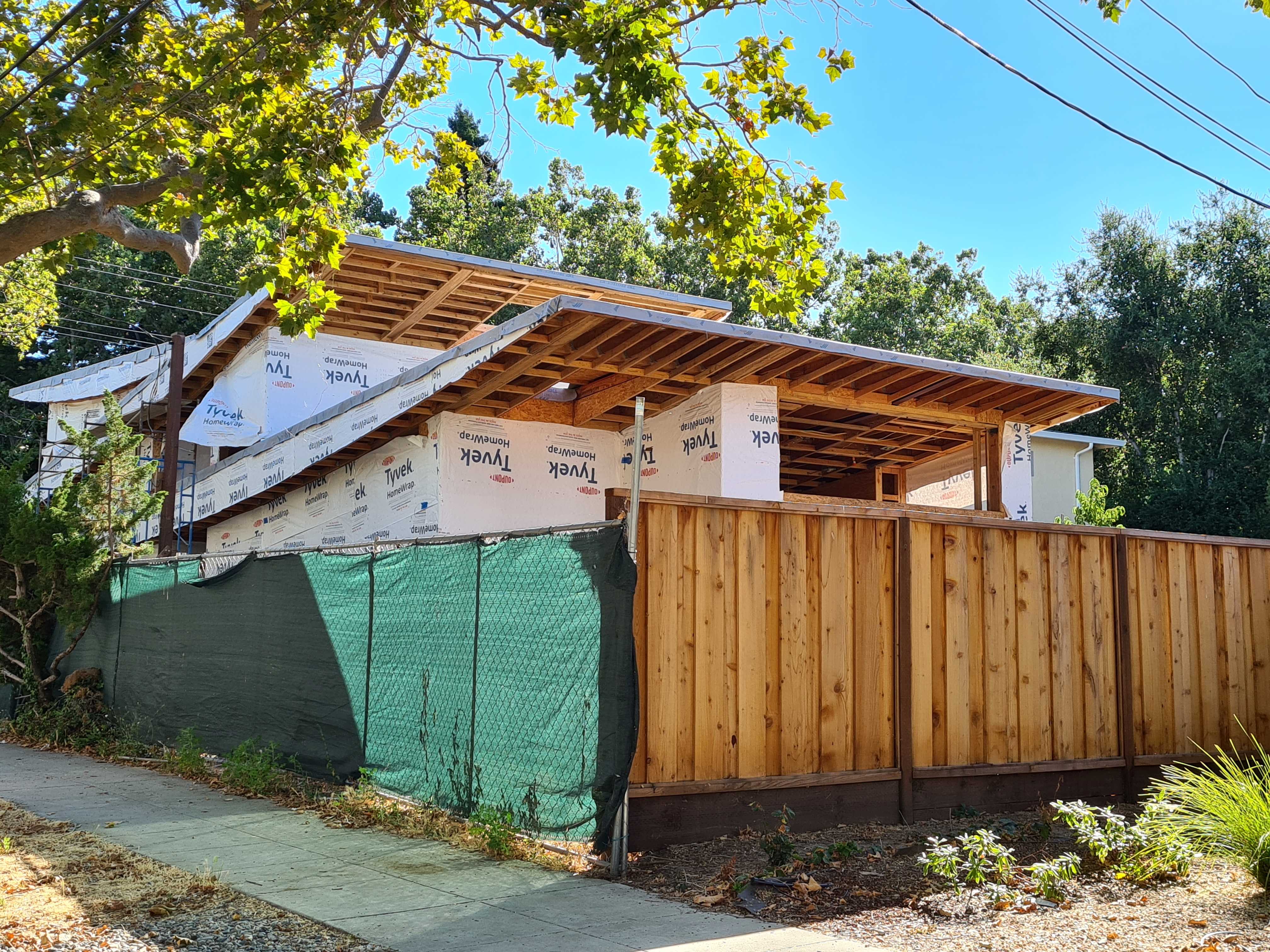
Energy Retrofits
Identify candidates for building energy retrofits, e.g., those with higher temperature sensitivity could use window upgrades or wall insulation.
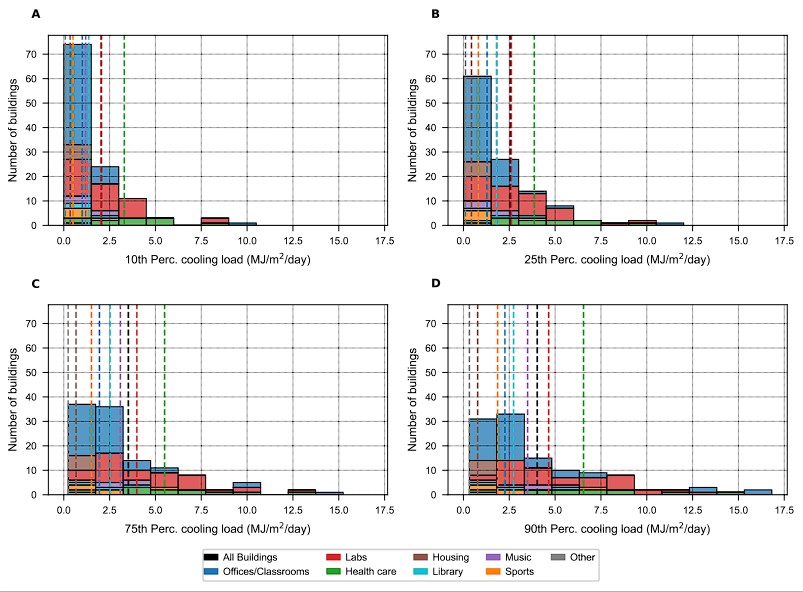
Fault Diagnosis
Flag any unusual behavior in buildings and assess whether mechanical retrofits and/or operational changes would be beneficial.
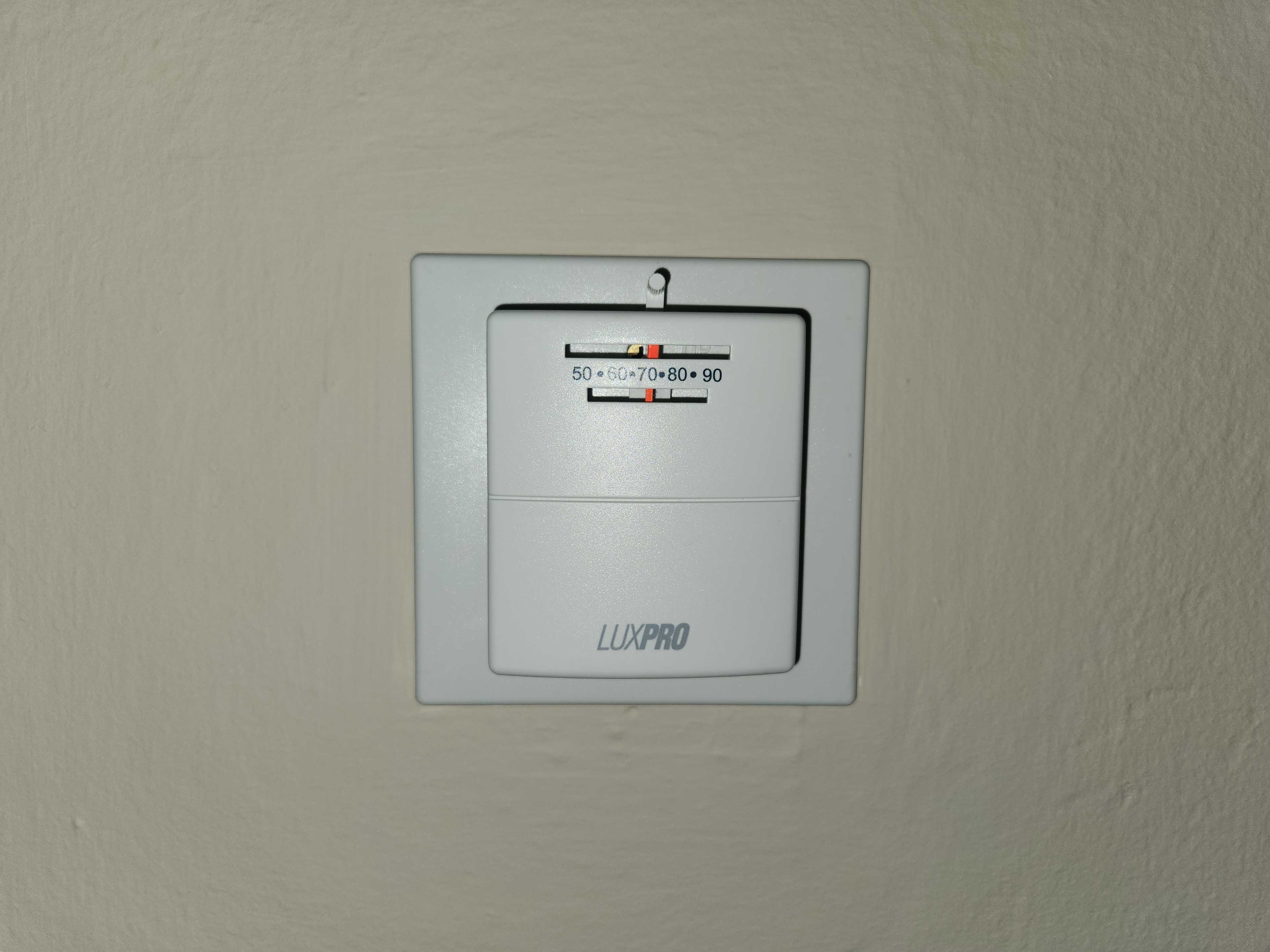
Demand Response
Assess the impacts e.g., reducing cooling in temperature sensitive buildings may cause substantial savings but reduces thermal comfort.
Ready to check out our insights?
Hit the button to go to our dashboard to view the data!